Harnessing Production Machine Learning for Enhanced Business Operations
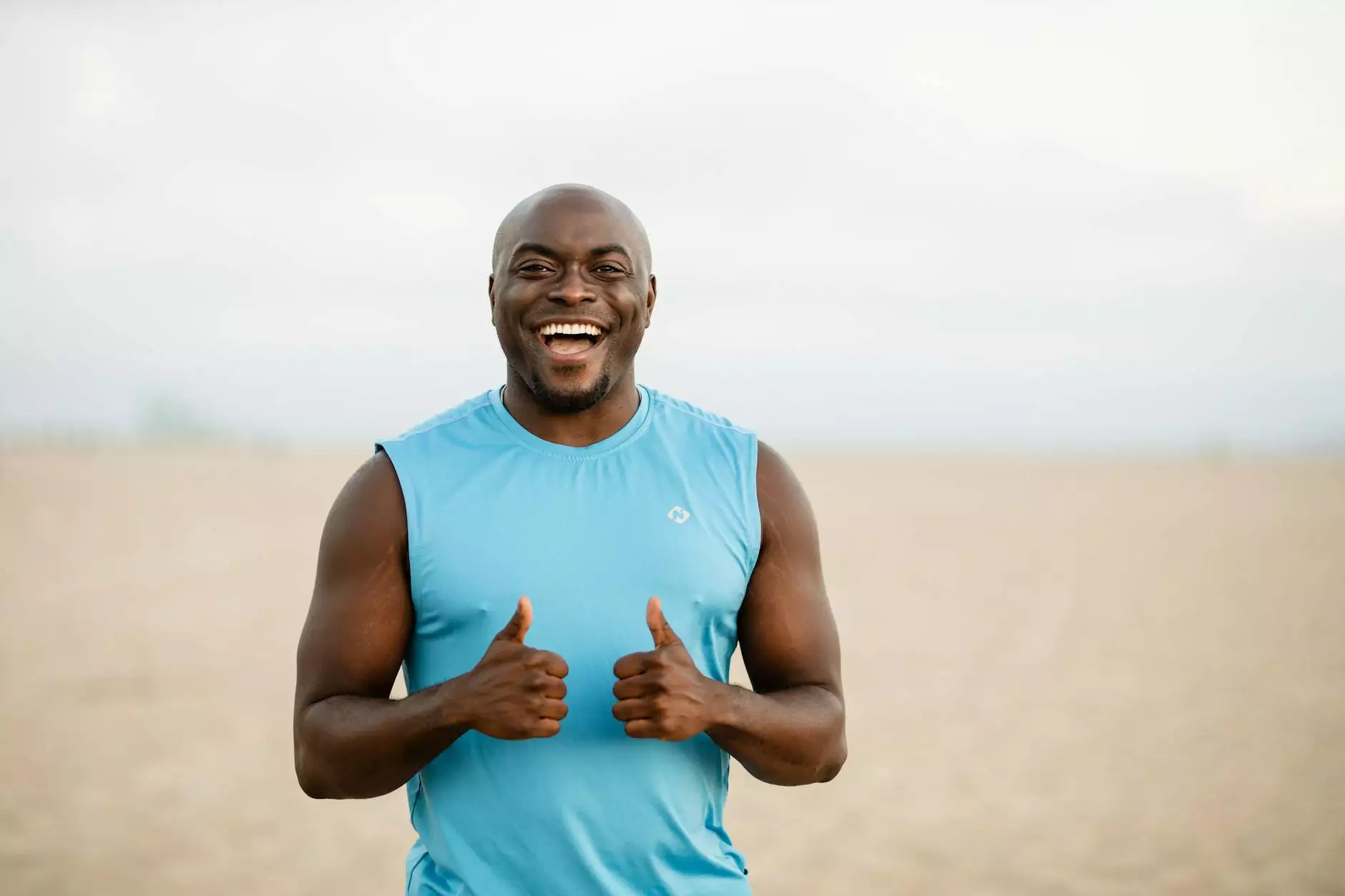
In today’s fast-evolving digital landscape, businesses are increasingly looking to leverage advanced technologies to stay ahead of the competition. One such technology that has gained significant traction is production machine learning. This powerful tool not only facilitates enhanced decision-making but also optimizes operational efficiencies across various industries. In this comprehensive article, we will explore the multifaceted benefits of production machine learning, especially within the domains of content management services, business process automation services, and data governance systems.
Understanding Production Machine Learning
Production machine learning refers to the use of machine learning models and algorithms in operational environments to enhance productivity and streamline processes. Unlike traditional machine learning, which may focus primarily on experimental or research settings, production machine learning emphasizes real-world applications and continuous improvement. This involves deploying trained models in production settings where they can automatically make predictions and offer insights based on real-time data.
The Importance of Production Machine Learning in Business
Incorporating production machine learning into business operations can yield numerous advantages:
- Increased Efficiency: Automating routine tasks and processes leads to a significant reduction in time and resources needed to complete operations.
- Improved Decision-Making: By analyzing data patterns and providing predictive insights, machine learning aids in informed decision-making based on empirical evidence.
- Scalability: As businesses grow, machine learning models can effortlessly scale to accommodate increased workloads and complexity.
- Cost Reduction: Automation and improved efficiencies lead to lower operational costs, maximizing profits.
Production Machine Learning Enhancing Content Management Services
In the realm of content management services, production machine learning plays a critical role in refining how businesses manage, distribute, and analyze their content. Here’s how:
1. Automated Content Curation
Machine learning algorithms can analyze user behavior and preferences to curate content that resonates with target audiences. This results in highly personalized experiences while reducing the time spent on manual content management.
2. Sentiment Analysis
Through natural language processing (NLP) capabilities, businesses can evaluate the sentiment of user-generated content, swiftly addressing negative feedback and amplifying positive responses. This proactive approach strengthens customer relationships and brand loyalty.
3. Performance Optimization
Utilizing machine learning, organizations can analyze the performance of various content types, determining what performs best in terms of engagement and conversions. This insight allows for informed adjustments to content strategies thereby maximizing effectiveness.
Streamlining Business Process Automation Services with Production Machine Learning
Business process automation services aim to streamline workflows and enhance productivity through automation. By integrating production machine learning into these services, businesses can:
1. Predict Maintenance Needs
Machine learning models can analyze historical data from machinery and equipment to predict maintenance requirements, thereby preventing costly downtimes and extending the lifespan of assets.
2. Automate Routine Tasks
From scheduling meetings to processing invoices, automation powered by machine learning can diminish the burden of repetitive tasks on employees, allowing them to focus on more strategic initiatives.
3. Enhance User Experience
With machine learning predicting user needs and automating responses, businesses can provide a seamless experience, which is crucial for customer satisfaction and retention.
The Role of Production Machine Learning in Data Governance Systems
Data governance is essential for any organization, ensuring data integrity, security, and compliance with regulations. Here’s how production machine learning enhances data governance systems:
1. Data Quality Management
Machine learning can identify anomalies and errors within datasets automatically, allowing businesses to maintain high standards of data quality without manual oversight.
2. Regulatory Compliance
By applying machine learning algorithms, organizations can monitor compliance-related activities continuously, flagging potential issues before they escalate into violations.
3. Enhanced Data Security
Machine learning can help detect fraud and unauthorized access by analyzing usage patterns and identifying suspicious activity, thereby fortifying data security measures.
Case Studies: Success Stories of Production Machine Learning
The benefits of adopting production machine learning are evidenced by numerous success stories across various industries. Here are a few notable examples:
1. Retail Industry
A leading e-commerce platform implemented production machine learning to analyze customer purchasing patterns. By doing so, they enhanced their recommendation engine, leading to a 25% increase in sales over six months.
2. Manufacturing Sector
A manufacturing company utilized machine learning for predictive maintenance, resulting in reduced downtime by 40% and savings on repair costs that significantly improved their bottom line.
3. Healthcare Domain
A healthcare provider leveraged machine learning to optimize patient management systems, identifying critical bottlenecks in their processes. This innovation improved patient care delivery times by 30%.
Challenges Facing Production Machine Learning Adoption
While the advantages of production machine learning are numerous, businesses must also navigate several challenges to successfully implement these technologies:
- Data Silos: Fragmented data can hinder machine learning initiatives. It’s crucial to have a centralized data ecosystem for effective model training.
- Initial Costs: The investment required for implementing machine learning infrastructure can be substantial, though the long-term benefits typically outweigh these costs.
- Talent Shortage: There is an ongoing demand for skilled professionals in the field of machine learning. Organizations may face difficulties in recruiting or training the right talent.
Best Practices for Implementing Production Machine Learning
To successfully implement production machine learning, businesses should consider the following best practices:
1. Define Clear Objectives
Before embarking on a machine learning project, organizations should set clear and measurable goals to evaluate success effectively.
2. Start Small
Implementing machine learning in smaller, manageable projects can mitigate risks and build momentum as successes are achieved.
3. Invest in Continuous Learning
The field of machine learning is constantly evolving. Continuous education for team members ensures that organizations remain at the forefront of technology.
4. Monitor and Optimize
Establishing a framework for monitoring the performance of machine learning models is critical for ongoing success. Regularly optimizing the models based on real-world feedback will enhance effectiveness.
The Future of Production Machine Learning
The future of production machine learning looks promising, with ongoing advancements in technology leading to even greater capabilities. Emerging trends include:
- Automated Machine Learning (AutoML): This empowers users with limited machine learning expertise to develop models easily, democratizing access to machine learning capabilities.
- Explainable AI (XAI): Growing emphasis on transparency in machine learning will drive solutions that provide explicable outputs for better trust and understanding.
- Integration with the Internet of Things (IoT): The synergy between IoT and machine learning will create real-time analytics opportunities, enhancing operational insights significantly.
Conclusion
The integration of production machine learning within business processes can lead to transformative changes, offering opportunities to increase efficiency, improve decision-making, and enhance overall performance. By leveraging machine learning technologies, organizations in the fields of content management services, business process automation services, and data governance systems can not only achieve operational excellence but also position themselves strategically for future growth in an ever-competitive market.
As businesses continue to embrace these advanced technologies, the potential for innovation and improvement in operations will become boundless. Embracing this journey towards a data-driven future is essential for any organization aiming for sustainable growth.