Understanding Semantic Segmentation in Video for Enhanced Data Annotation
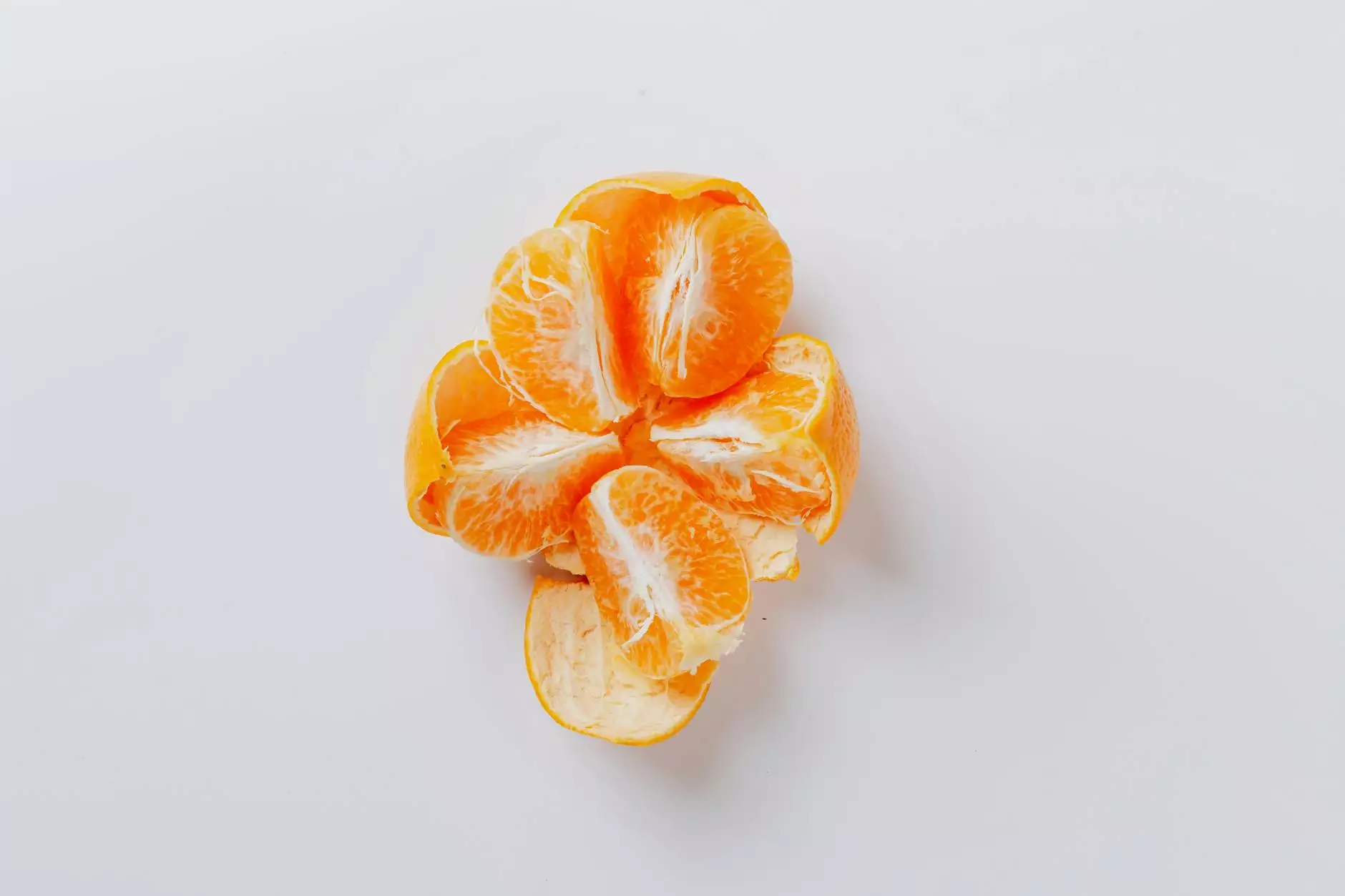
In the world of data annotation, semantic segmentation video plays a crucial role in allowing machines to understand visual content with remarkable accuracy. This process involves dividing a video into multiple segments, where each segment corresponds to a distinct object or region within the visual frame. It empowers artificial intelligence applications, leading to improved performance in tasks such as object detection, image recognition, and video analysis.
What is Semantic Segmentation?
Semantic segmentation refers to the process of categorizing each pixel of an image or video frame into predefined classes. This technique is essential in various applications, including autonomous driving, medical image analysis, and robotic perception. By labeling every pixel, semantic segmentation creates a detailed outline of objects for machine learning models, enabling them to make sense of complex visual data.
The Importance of Video in Semantic Segmentation
When it comes to working with video data, semantic segmentation becomes even more critical. Videos capture motion and temporal dynamics which static images do not provide. This additional temporal component introduces new challenges, such as:
- Increased complexity due to varying object appearances and motions.
- The need for temporal coherence, ensuring that segmentation remains consistent across frames.
- Handling occlusions where objects may be blocked by others, complicating segmentation tasks.
Applications of Semantic Segmentation in Video
Understanding the applications of semantic segmentation can illuminate why it matters so much in today’s digital landscape. Here are some significant areas where this technology is applied:
1. Autonomous Driving
In the realm of autonomous vehicles, semantic segmentation assists in identifying road signs, pedestrians, other vehicles, and lane markings in real-time. This capability helps ensure safety and efficiency in driving algorithms.
2. Video Surveillance
Semantic segmentation can improve the accuracy of surveillance systems by enabling better recognition and tracking of individuals and objects across multiple video feeds. Such capabilities enhance security measures and threat detection.
3. Medical Imaging
In healthcare, semantic segmentation of videos, such as ultrasound or MRI scans, aids in the diagnosis by clearly identifying relevant anatomical structures and pathological changes.
4. Interactive Gaming and VR
In the gaming industry, semantic segmentation helps developers create immersive experiences by allowing the game engine to understand the environment and the various objects within it, leading to more interactive and engaging gameplay.
Challenges in Semantic Segmentation of Video
While semantic segmentation has immense potential, it also comes with challenges:
- Data Quality: The quality of the training data significantly affects the model's performance. Inconsistent labeling or low-quality footage can lead to inaccurate segmentations.
- Computational Demand: Segmenting videos requires substantial computational resources, particularly with high-resolution videos.
- Scalability: As the volume of video data increases, maintaining high accuracy without compromising performance becomes more challenging.
Leveraging KeyLabs.ai for Data Annotation
At KeyLabs.ai, we recognize the importance of well-annotated data for training high-performance AI models. Our data annotation tool specializes in enabling semantic segmentation video, allowing our clients to annotate their video datasets with exceptional accuracy.
Features of KeyLabs.ai Data Annotation Platform
- User-Friendly Interface: Our platform is designed for ease of use, ensuring that annotators can work quickly and efficiently without a steep learning curve.
- Automated Annotation Tools: Using advanced algorithms, KeyLabs.ai offers automated annotation features that assist human annotators, significantly speeding up the process while maintaining high accuracy.
- Quality Control: We implement rigorous quality control measures to ensure that annotations meet industry standards, including regular audits and revisions.
- Scalability: Our platform is built to handle large volumes of data, making it suitable for projects of all sizes—from small datasets to extensive video libraries.
Steps to Annotate Semantic Segmentation in Video with KeyLabs.ai
By choosing KeyLabs.ai, annotating videos for semantic segmentation becomes a streamlined process. Here’s how:
- Upload Your Video: Start by uploading your video datasets directly to our platform.
- Define Annotation Guidelines: Clearly establish the classes for segmentation (e.g., cars, people, bicycles).
- Begin Annotation: Utilize our intuitive tools to annotate each frame of the video accurately.
- Review and Refine: Leverage our quality control features to review annotated segments, making adjustments as necessary.
- Export Your Annotations: Once satisfied, export your annotated datasets for use in training your AI models.
Conclusion
In conclusion, the role of semantic segmentation video is a cornerstone in the field of data annotation, fundamentally enhancing the capabilities of AI systems in various applications. With the ongoing evolution of visual data analytics, platforms like KeyLabs.ai stand out as essential allies in delivering accurate and efficient annotation solutions. By leveraging our state-of-the-art data annotation tools, businesses can ensure their AI models are trained on high-quality, well-annotated datasets that ultimately drive innovation and intelligence in their operations.
Investing in proper semantic segmentation practices today will pave the way for more advanced machine learning capabilities tomorrow. Embrace the future of data annotation with KeyLabs.ai — where quality and precision are at the forefront of every project.