Unlocking Business Potential with Image Annotation for Machine Learning
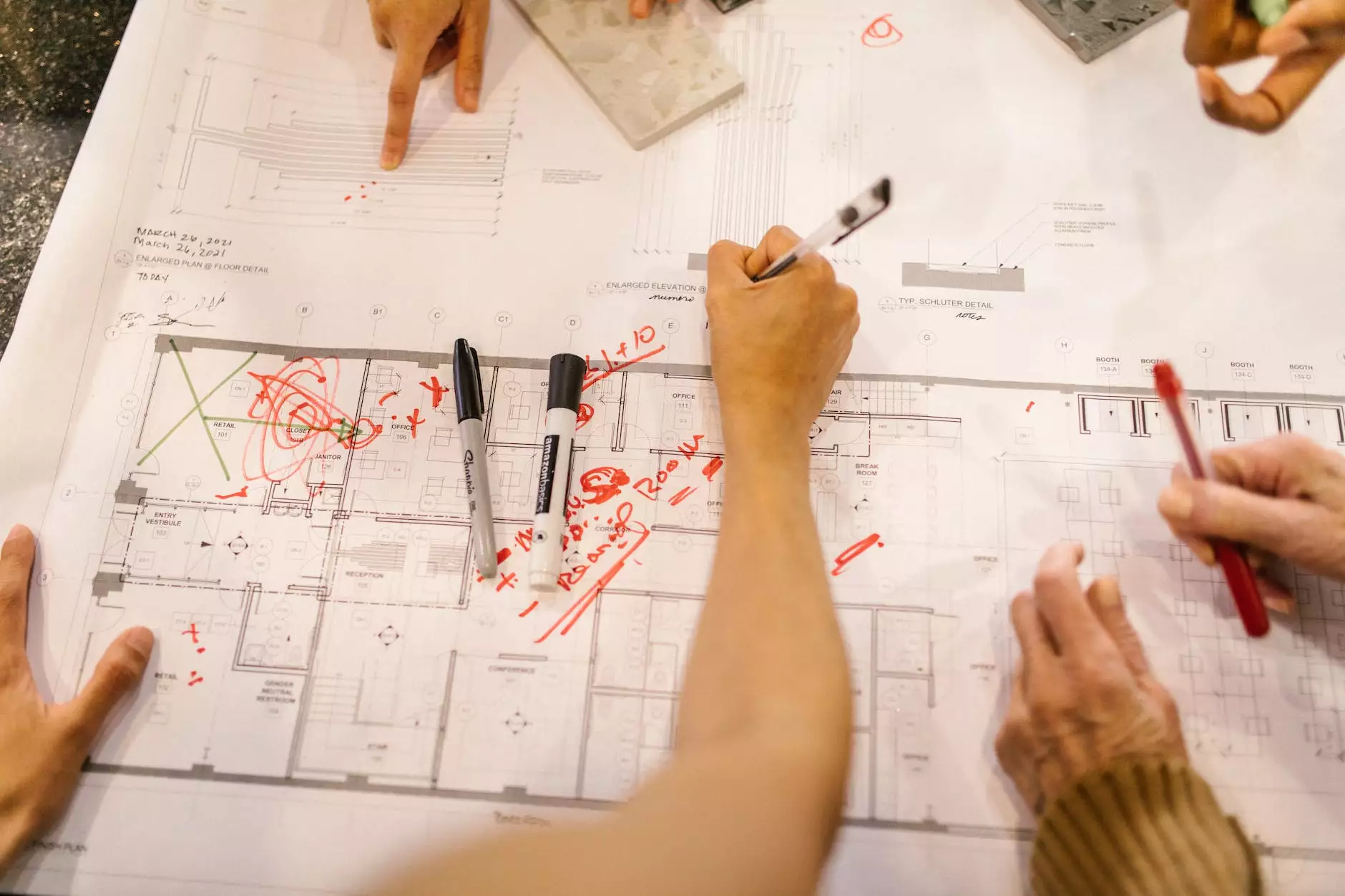
The integration of technology into business processes has revolutionized how companies operate and serve their customers. For industries such as Home Services and Keys & Locksmiths, the utilization of cutting-edge technologies has become essential to stay competitive and relevant in the market. One such transformative technology is image annotation for machine learning, which serves as a pivotal tool in enhancing operational efficiency and customer satisfaction.
Understanding Image Annotation for Machine Learning
Image annotation involves labeling images to help machines understand the content within them. In the realm of machine learning, this process is crucial as it allows algorithms to learn from accurately labeled datasets. The goal is to train systems to recognize objects, scenes, and even textual information in images. This technique is fundamentally important for various applications, including computer vision, automated image recognition, and self-driving technologies.
The Importance of Image Annotation
As businesses evolve, the significance of image annotation for machine learning cannot be overstated. Here are some key reasons why businesses, especially those in the Home Services and Locksmith sectors, should prioritize this technology:
- Enhanced Decision Making: By analyzing visual data, businesses can make informed decisions that lead to better outcomes.
- Improved Customer Experience: Accurate image recognition can streamline service delivery and enhance customer interactions.
- Increased Efficiency: Automating processes through machine learning can significantly reduce operational costs and time.
- Data-Driven Strategies: Collecting and annotating images creates vast datasets that can be analyzed for insights and trends.
Applications of Image Annotation in Business
The application of image annotation for machine learning spans across various domains. For the Home Services industry, it can be utilized for tasks such as:
1. Property Inspection and Maintenance
In sectors such as real estate and property management, annotated images can assist in conducting detailed inspections. Algorithms can be trained to identify maintenance issues, assess property conditions, and recommend necessary repairs through visual data analysis. This leads to proactive maintenance strategies that save time and resources.
2. Inventory Management for Locksmiths
Locksmiths can leverage image annotation to manage their inventory more effectively. By using annotated images of keys and locks, machine learning models can predict inventory needs, track availability, and even automate reordering processes, thereby improving service efficiency.
3. Customer Relationship Management
Enhancing customer interactions through personalized services is crucial. Image annotation allows businesses to analyze customer submissions (photos of locks, keys, etc.) and provide tailored solutions. This not only enhances customer satisfaction but also builds brand loyalty.
The Process of Image Annotation
Understanding the process of image annotation is vital for businesses looking to implement this technology effectively. Here are the key steps involved:
1. Data Collection
The first step is gathering a sufficient amount of images relevant to the business. For locksmiths, this could mean images of various types of keys and locks from different angles.
2. Annotation
This step involves labeling the images accurately. For example, indicating which parts of a lock are visible in the image, categorizing them by type, or marking key patterns. This can be done using annotation tools, which may include bounding boxes, polygons, or semantic segmentation techniques.
3. Model Training
Post-annotation, the labeled data is utilized to train machine learning models. The better the quality of the annotations, the more accurate the model will be, resulting in a high-performing artificial intelligence system.
4. Evaluation
Once the model is trained, it needs to be evaluated for accuracy and reliability. This involves running tests with new images that the model hasn’t seen before.
Challenges in Image Annotation
While the potential of image annotation for machine learning is vast, there are challenges that businesses may face:
- Time-Consuming: The process of annotating images can be labor-intensive and require a considerable time investment.
- Quality Control: Ensuring the accuracy of annotations is crucial. Poorly labeled data can lead to ineffective model training.
- Scalability: As businesses grow, so does the volume of images that need to be annotated. Scaling the annotation process can be complex and resource-heavy.
Best Practices for Successful Image Annotation
To overcome these challenges and harness the full power of image annotation for machine learning, businesses can adopt several best practices:
1. Utilize Advanced Tools
Making use of state-of-the-art annotation tools can streamline the process, offering features such as auto-annotation and collaboration options for teams.
2. Employ Experienced Annotators
Having skilled annotators who understand the nuances of your industry will significantly improve the quality of the labeled data.
3. Continuous Learning and Model Improvement
The machine learning landscape is always evolving. It is important for businesses to continually update their models with new data to improve accuracy and performance.
Real-World Examples
Across various industries, many organizations have effectively utilized image annotation for machine learning:
Example 1: Real Estate Platforms
Real estate platforms that use image annotation can automatically detect features in homes, categorize properties, and enhance search functionality based on annotated images, thus improving user experiences.
Example 2: Automotive Industry
In the automotive sector, companies employing image annotation for machine learning have accelerated the development of self-driving cars. They annotate images of vehicles, road signs, and obstacles to train their autonomous systems.
Future Trends in Image Annotation
The future of image annotation for machine learning holds exciting possibilities:
- Increased Automation: As machine learning progresses, we can expect a rise in fully automated annotation processes.
- Real-Time Annotation: Innovations in technology may allow for real-time image annotation, enhancing immediacy in applications.
- Integration with Augmented Reality (AR): Merging image annotation with AR could lead to more immersive customer experiences in the locksmith industry.
Conclusion
In conclusion, image annotation for machine learning is not merely a technical necessity but a strategic enabler for businesses, especially those in the Home Services and Keys & Locksmiths sectors. By embracing this technology, companies can significantly improve their operations, enhance customer satisfaction, and stay ahead in an increasingly competitive landscape. Adopting best practices in the annotation process, addressing challenges proactively, and continuously evolving with technology will position businesses for success in the future.
Investing in image annotation solutions is not just an operational decision; it's a step towards innovation and excellence. As the world continues to rely more heavily on visual data, equipping your business with the tools and knowledge to leverage image annotation will unlock new opportunities and drive unparalleled growth.